The Dark Side of Predictive Analytics: Mitigating Ethical Risks in Online Marketing

Related Posts

Deepfakes and Ethical Boundaries: Protecting Brands in Online Marketing
The Rise of Synthetic Media and Its Impact on Online Marketing The internet has forever changed the way we connect, communicate, and consume information. With the advent of synthetic media, online marketing is undergoing a monumental shift. Synthetic media refers...
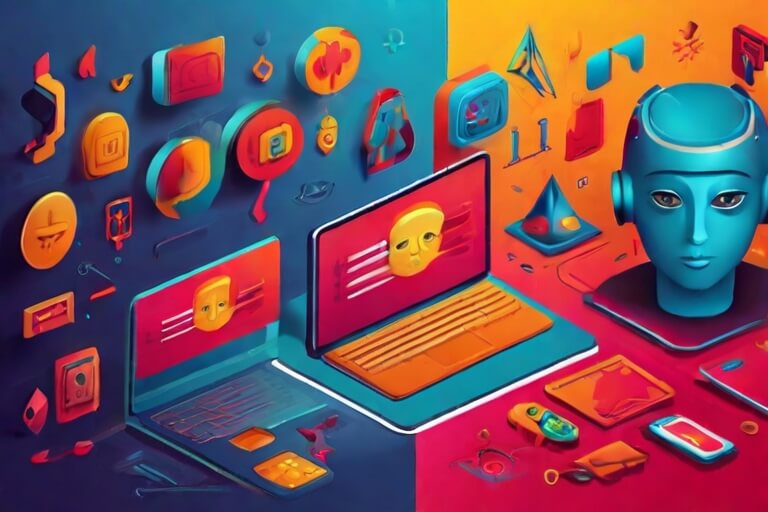
Ethical Implications of AI Chatbots in Customer Engagement for Online Brands
The Influence of AI Chatbots on Customer Engagement for Online Brands As the advancement of artificial intelligence (AI) continues to reshape various industries, online brands have started to leverage AI chatbots to enhance their customer engagement strategies. AI chatbots are...

The Future of Ethical AI Guidelines: Shaping Responsible Practices in Online Marketing
Understanding Ethical AI Guidelines in Online Marketing Online marketing has revolutionized the way companies promote their products and reach out to potential customers. With the advent of artificial intelligence (AI), marketers now have access to powerful algorithms that can analyze...
Table of Contents
hide